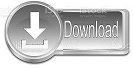
Please,” will it continue to work?įinally, and perhaps most perversely, some of the most predictive variables are circular.įor example, in another paper, the computer scientists Lars Backstrom, Jon Kleinberg, Lillian Lee and Cristian Danescu-Niculescu-Mizil predict which posts on Facebook generate many comments. But if everyone starts asking you to “Share this article. In the Twitter example, the use of the words “retweet” or “please” were predictive. Calling a food “artisanal” was eye-catching, until it became so common that we’re not far away from an artisanal plunger. When few people do something, it catches the eye when everyone does it, it is ho-hum. But once an algorithm finds those things that draw attention and starts exploiting them, their value erodes. Rarity and novelty often contribute to interestingness - or at the least to drawing attention. So the lesson is not “make your tweets longer” but “have more content,” which is far harder to do.Īnother problem comes from an inherent paradox in predicting what is interesting.
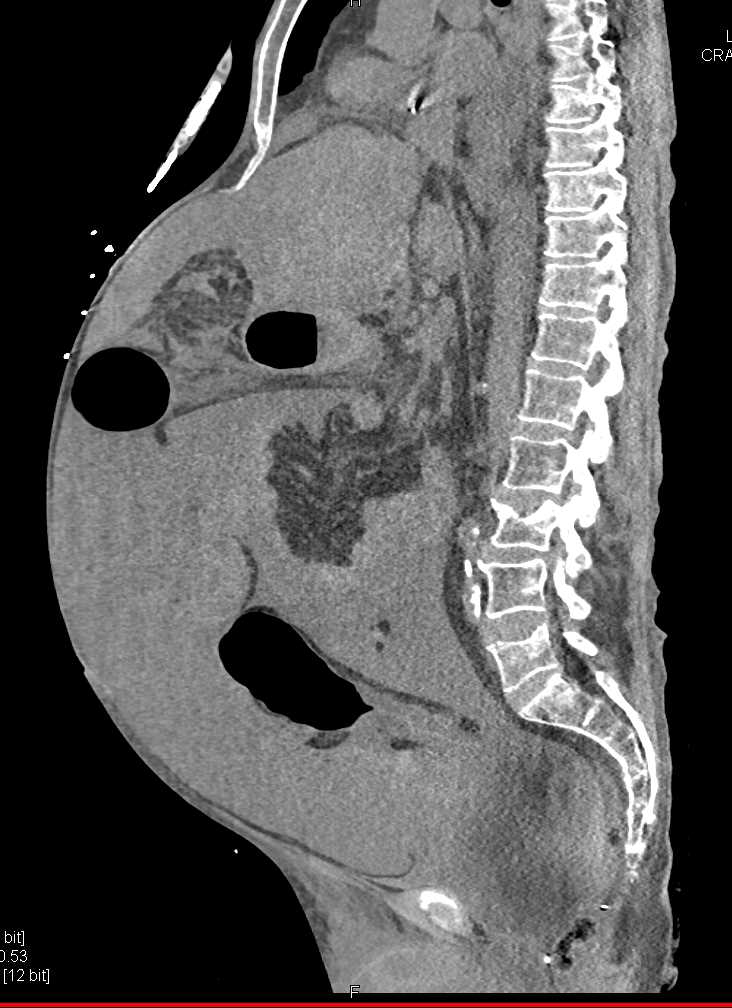
Instead, length is probably a good predictor because longer tweets have more content. The old adage that “less is more” is, if anything, truer in this medium. It seems unlikely that you should therefore write longer tweets. For example, the tweet predictor finds that longer tweets are more likely to be retweeted. The causality problem can show up in very subtle ways. It can guess which tweet gets retweeted about 67 percent of the time, beating humans, who on average get it right only 61 percent of the time. The end result is an algorithm that guesses well. This is usually how “smart” algorithms are created from big data: Large data sets with known correct answers serve as a training bed and then new data serves as a test bed - not too differently from how we might learn what our co-workers find funny. It used a data set of around 11,000 paired tweets - two tweets about the same link sent by the same person - to learn which word patterns looked predictive and then tested whether these patterns hold in new data. To see why, it is useful to see how the algorithm was built. That an algorithm can make these kinds of predictions shows the power of “big data.” It also illustrates a fundamental limitation of big data: Specifically, guessing which tweet gets retweeted is significantly easier than creating one that gets retweeted. (The answer: Gore’s first tweet got more retweets). You can think of the pair of Gore tweets as a practice round for a 25-question quiz that The Upshot has created based on their algorithm. Three computer scientists, Chenhao Tan, Lillian Lee and Bo Pang, have built an algorithm that also makes these guesses, as described in a recent paper, and the results are impressive.
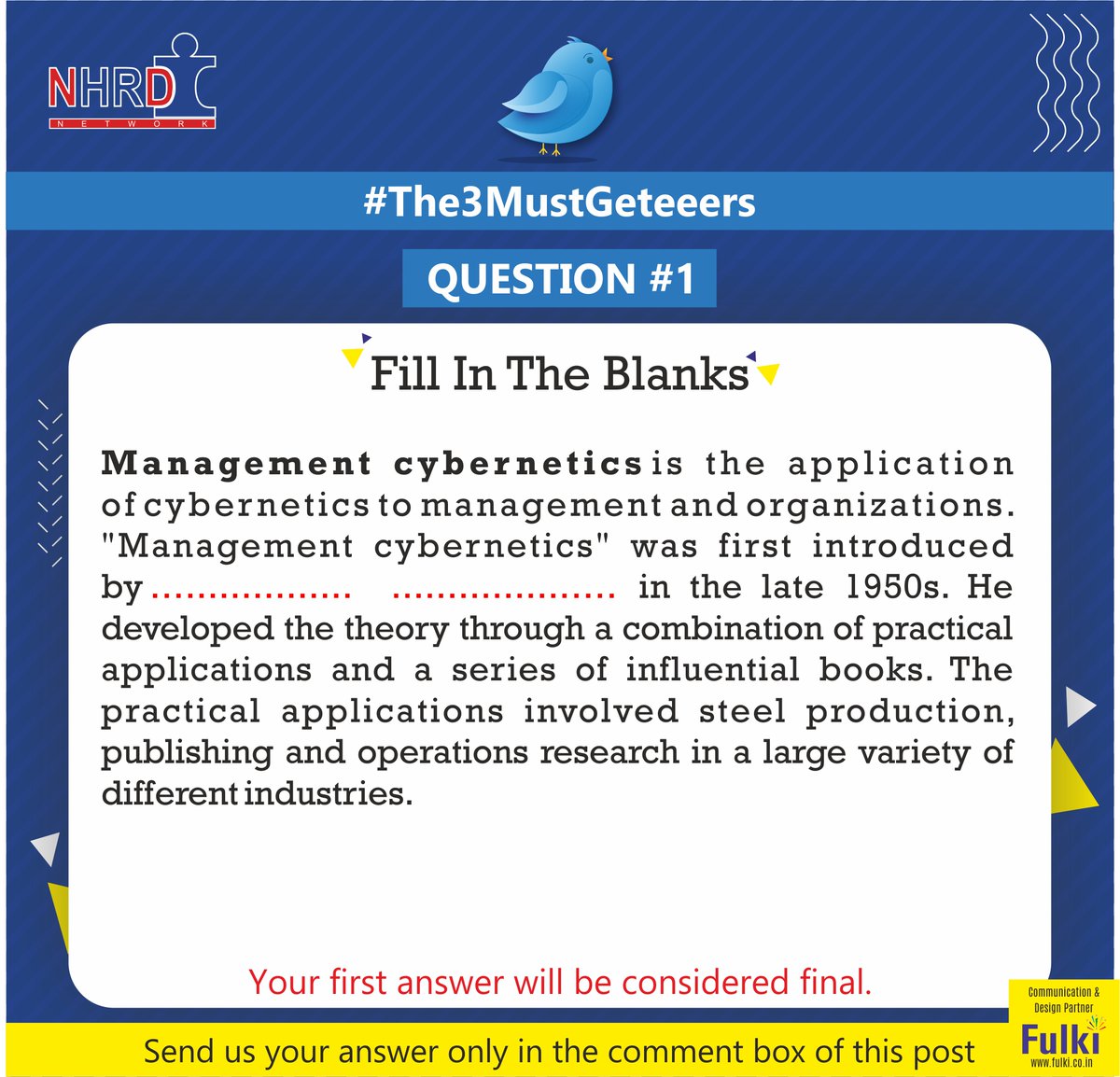

Can you guess which one was retweeted more often?
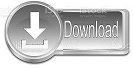